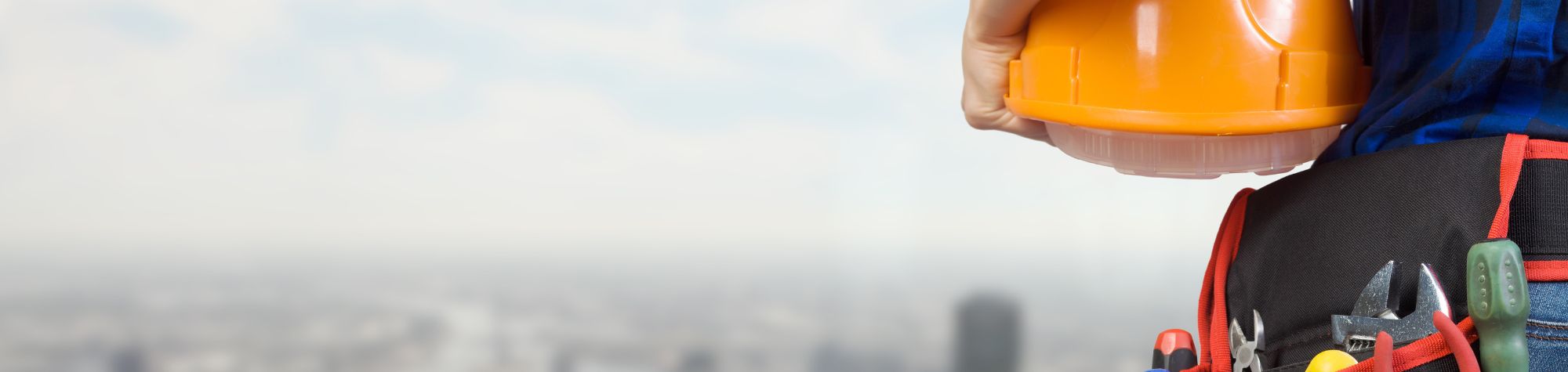
Artificial intelligence (AI) is rapidly transforming manufacturing—from assembly lines to warehouse floors—by streamlining operations, boosting productivity, and reducing costs. According to Building Radar’s guide on AI in manufacturing, manufacturers that adopt AI-driven tools for early anomaly detection report up to 30% fewer unplanned stoppages. Meanwhile, cross-industry insights from OpenAsset’s overview on AI in construction demonstrate how similar machine-learning techniques help detect wear and tear on heavy equipment before failures occur.
Beyond maintenance, AI adoption in manufacturing unlocks smarter process automation and real-time quality control. A recent ConstructConnect report highlights how data-driven models can optimize production schedules and dynamically reroute workflows when machine capacity shifts. By applying these strategies on the factory floor, companies reduce idle time, tighten cost controls, and deliver higher-quality products at scale.
What AI adoption looks like on the factory floor
Understanding AI’s role in modern production
At its core, AI mimics human decision-making by analyzing vast datasets in milliseconds. In manufacturing, this translates to systems that learn from sensor feeds—temperature, vibration, pressure—and spot patterns invisible to the naked eye. Over time, machine learning models refine their predictions, making them more accurate at forecasting breakdowns or quality defects.
Process automation with intelligent robotics
Robotic arms have long handled repetitive tasks like welding or material placement. AI takes this further by giving robots vision systems to inspect parts and adaptive control to adjust grip pressure or speed. For example, AI-driven pick-and-place robots can differentiate between slightly warped products, ensuring only components within tolerance move to the next station.
Automating workflows for efficiency
Dynamic scheduling and flow control
Traditional production schedules assume machines run 100% of the time—an assumption shattered by real-world hiccups. AI systems monitor machine health and material availability, automatically reshuffling job sequences to avoid bottlenecks. If one line slows due to tooling maintenance, the software routes work to alternate cells, maximizing uptime.
Reducing manual data entry and paper trails
Many factories still rely on paper logs, barcode scans, and spreadsheets to track output. AI-powered digitization tools convert handwritten notes into structured data, flag discrepancies, and update enterprise resource planning (ERP) systems in real time. This not only eliminates transcription errors but also frees staff to focus on higher-value tasks.
Predictive maintenance for uninterrupted operation
Spotting failures before they happen
Sensors on bearings, motors, and conveyors feed AI engines that recognize early warning signs—unusual vibration patterns or heat spikes. By scheduling maintenance only when needed, rather than on a fixed calendar, manufacturers cut service costs by up to 20% and avoid surprise downtime.
Extending asset lifecycles
Predictive algorithms calculate remaining useful life for critical components. Maintenance teams receive prioritized work orders, ensuring parts are serviced at the optimal moment. Over months, data shows fewer premature replacements and improved return on equipment investments.
Elevating quality control with computer vision
Automated defect detection
High-resolution cameras, paired with convolutional neural networks (CNNs), inspect every part in milliseconds. They catch minute cracks, surface blemishes, or assembly faults that human eyes might miss at high line speeds. AI systems learn from both accepted and rejected parts, continuously improving inspection accuracy.
Root-cause analysis for continuous improvement
When defects occur, AI correlates quality data with upstream processes—machine settings, material batch numbers, or operator actions. This helps engineers pinpoint the true origin of issues and implement corrective actions swiftly, reducing scrap rates over time.
Optimizing supply chains and inventory
Demand forecasting and replenishment
AI models analyze historical sales, seasonality, and external factors—like raw-material price swings—to predict future demand. Integrated with inventory management systems, they trigger purchase orders just in time, preventing stockouts and minimizing holding costs.
Supplier risk assessment
Machine learning scours news feeds, credit reports, and shipping logs to flag supply-chain disruptions—natural disasters, labor disputes, or geopolitical events. Procurement teams receive alerts when a critical component supplier faces potential delays, allowing them to source alternatives proactively.
Customization at scale
Mass personalization with flexible lines
Consumers increasingly expect tailored products—whether custom colors, components, or packaging. AI-driven manufacturing execution systems (MES) translate individual orders into specific machine instructions on the fly. Lines reconfigure automatically, switching tools or adjusting parameters to fulfill unique configurations without manual intervention.
Virtual simulations for design validation
Before cutting steel or molding plastic, AI-based digital twins replicate entire production lines in a virtual environment. Engineers test new product variants, validate tooling changes, and estimate cycle times—eliminating physical prototyping costs and speeding up time to market.
Strengthening workplace safety and compliance
Real-time hazard detection
Computer vision cameras monitor factory floors, identifying unsafe behaviors—workers without safety gear or vehicles entering restricted zones. AI systems send instant alerts, reducing accident rates and ensuring compliance with OSHA standards.
Environmental monitoring and reporting
Sensors for air quality, noise, and chemical exposure feed AI dashboards that track regulatory limits. When thresholds approach danger, automated shut-offs or exhaust controls engage. Detailed logs simplify audits and prove compliance to authorities.
Cross-Industry Innovation: Parallels with Construction AI
While manufacturers harness AI for predictive maintenance and automated inspections, the construction industry applies similar techniques to project tracking and early opportunity spotting. Just as AI in manufacturing predicts machine health, Building Radar’s AI sifts through global data sources to flag new construction projects at the planning phase. This early-warning system empowers sales teams with first-mover advantage—much like predictive maintenance keeps production lines running smoothly.
Moreover, the qualification checklists and mobile-friendly templates that guide field teams in construction mirror the digital twin simulations and workflow automations on factory floors. Both industries benefit when AI surfaces actionable insights—whether it’s a potential bearing failure or a permit filing for a large-scale build—allowing organizations to allocate resources proactively and maintain continuous operations.
Harnessing AI Insights Together: Building Radar Meets Manufacturing
Building Radar’s scalable Revenue Engineering Software demonstrates how AI-driven intelligence transforms both project sales and industrial operations. By filtering over 45 search criteria—region, builder, project size—construction professionals never miss relevant opportunities. Similarly, manufacturing teams can adopt configurable AI filters to monitor equipment metrics, production KPIs, and supply-chain alerts. The result: a unified approach to early detection, whether spotting a high-margin construction contract or preventing an unexpected line stoppage.
Seamless CRM integrations with platforms like Salesforce and HubSpot keep every lead and maintenance alert in one place. Automated outreach templates in sales mirror the work-order templates in maintenance management systems, standardizing responses and follow-ups. Dedicated Customer Success Managers for Building Radar ensure best practices are shared—parallel to how industrial AI consultants guide factories through pilot projects and scale-up phases.
Charting the Future of Intelligent Manufacturing
From smart robots that adapt in real time to sensors that predict failures before they occur, AI’s potential in manufacturing is vast—and only just beginning. Companies that embrace AI adoption, process automation, and predictive maintenance will outpace competitors in quality, cost control, and agility. Drawing on cross-industry innovations like Building Radar’s project-identification AI, manufacturers gain a roadmap for integrating advanced analytics, dynamic workflows, and digital twins into their operations. The factories of tomorrow will be safer, cleaner, and more efficient—powered by intelligent systems that learn, adapt, and drive continuous improvement.
Relevant Links
- How AI can be used in manufacturing (Building Radar Blog)
- Building Radar
- Building Radar Insights
- Building Radar Features
- Building Radar Construction Projects
- Building Radar Tenders
- Building Radar Reference Customers
- How to use AI in construction (OpenAsset)
- AI in construction has landed (ConstructConnect)
- The rise of artificial intelligence in construction (Construction Today)
- AI solutions for construction engineering (Oracle)